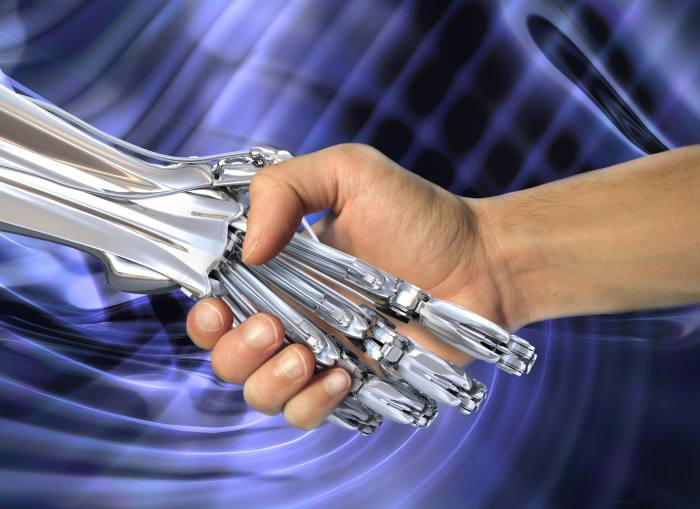
New examples of how artificial intelligence can help financial advice seem to emerge almost every week.
A new service from Mercer, which will help defined benefit schemes understand which members are most likely to accept opt-out offers so they can manage liquidity and risk, is the latest example.
Using anonymised data from similar previous exercises and the scheme’s own data, a machine-learning algorithm determines the probability of a member accepting an offer.
The system provides a dashboard of expected outcomes, based on differing offers and demographics. This can build in a wide range of efficiencies for consultants and the actuaries.
For example, it enables them to provide instant answers to the inevitable 'what if' questions that are bound to come from clients in such situations.
Employers and trustees get far quicker answers, thus removing the need for extra meetings. Mercer can also send the client a link to the dashboard so they can then explore the options in their own time.
Using a data-driven approach helps pension schemes and sponsors better manage risk through planning member options projects that have optimum member offer structures.
The tool has identified several factors affecting a member’s transfer out decision. These include age, location and the time of year.
The service has been subject to extensive back-testing before going live and some data was kept out in order to have a control set. This is a crucial area of due diligence in any AI system.
Also, there are built-in anti-bias tests to ensure no historic biases are maintained.
In reality, all human processes have a risk of bias, which machines can potentially help us to identify and remove.
Mercer found when they removed certain data the results got more accurate. I would expect this service to be very attractive to employers with DB schemes.
Ian McKenna is founder and director at FTRC